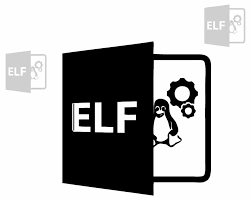
Understanding the ELF Object File Format: A Look Back and Beyond
The ELF object file format is widely adopted by many UNIX-like operating systems. Exploring the historical evolution of ELF and its connection to the System V ABI offers interesting insights. The ELF format comprises the generic specification, processor-specific specifications, and OS-specific specifications. Three key documents often emerge when searching for the generic specification: The TIS…
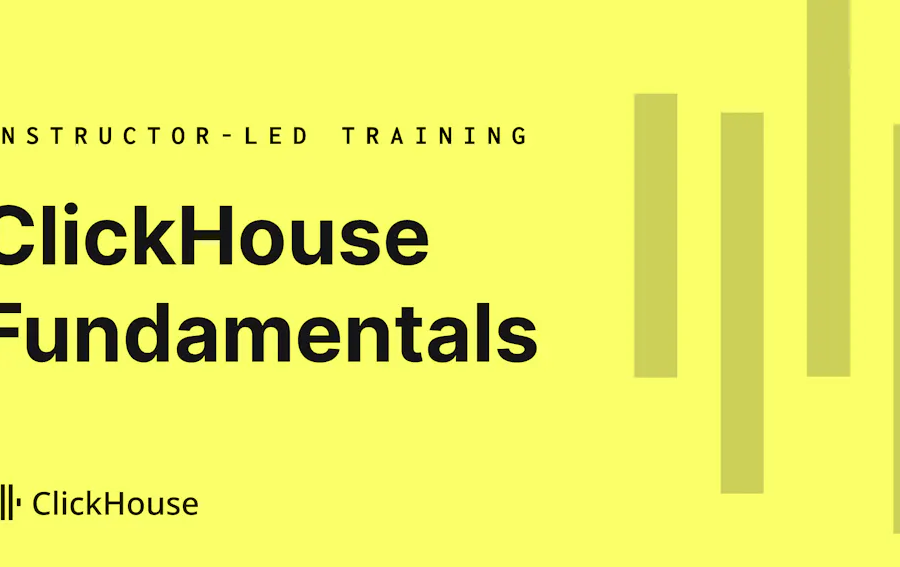
ClickHouse Fundamentals for Free: Master the High-Performance Database
Have you heard the buzz about ClickHouse, the ultra-fast analytical database engine? Curious to see what it can do for your data analysis needs? We’ve got the perfect opportunity for you! Free ClickHouse Fundamentals Training We’re offering a comprehensive free 2-day (3 hours each day) instructor-led training program, “ClickHouse Fundamentals.” This is your chance to…
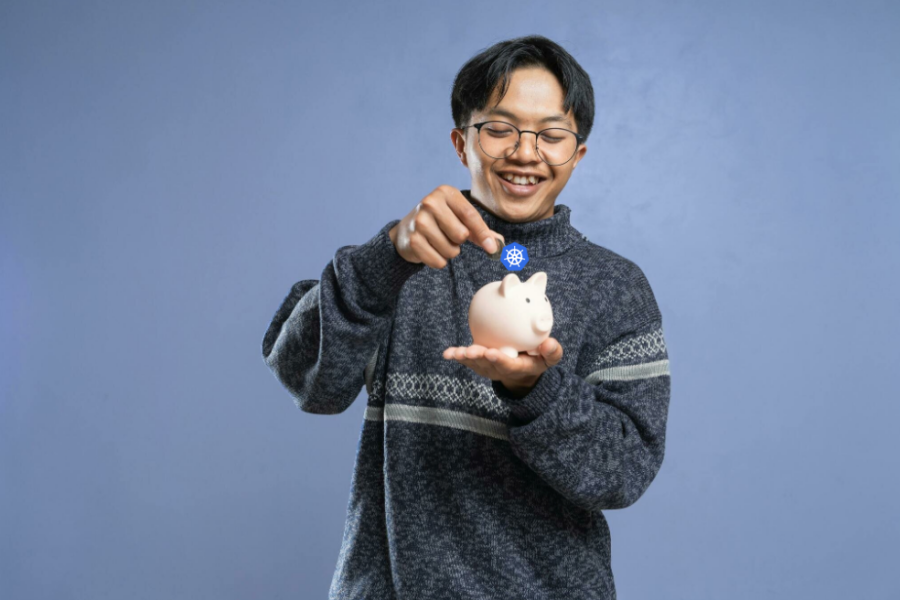
Free Webinar: Master Kubernetes Cost Optimization on AWS
Cloud-native environments offer incredible flexibility and scalability, but managing costs can be a constant battle. This free webinar, brought to you by AWS, Kubecost, and Nirmata, will equip you with the knowledge and tools to prevent cost overruns before they happen. The Power of Proactive Cost Control This webinar dives deep into a powerful combination:…
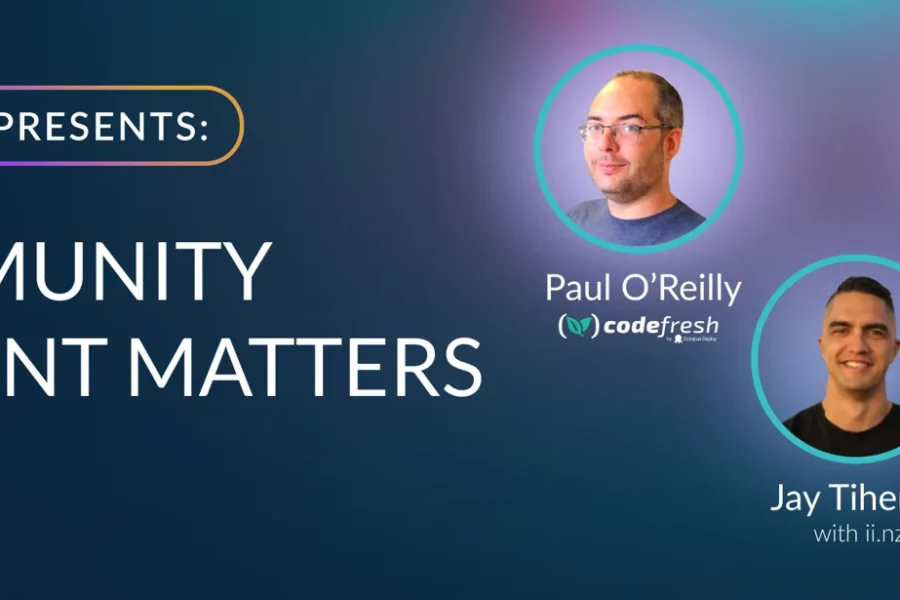
Collaborate and Thrive: The Essential Role of Community Involvement
Date : June 12Time : 2-3 pm PST Why Community Involvement is Crucial Community involvement is a cornerstone of personal and professional development. Engaging with the community provides numerous benefits, ranging from personal growth to advancing one’s career. For individuals in the STEM fields, as well as adult engineers, community engagement fosters a sense of…
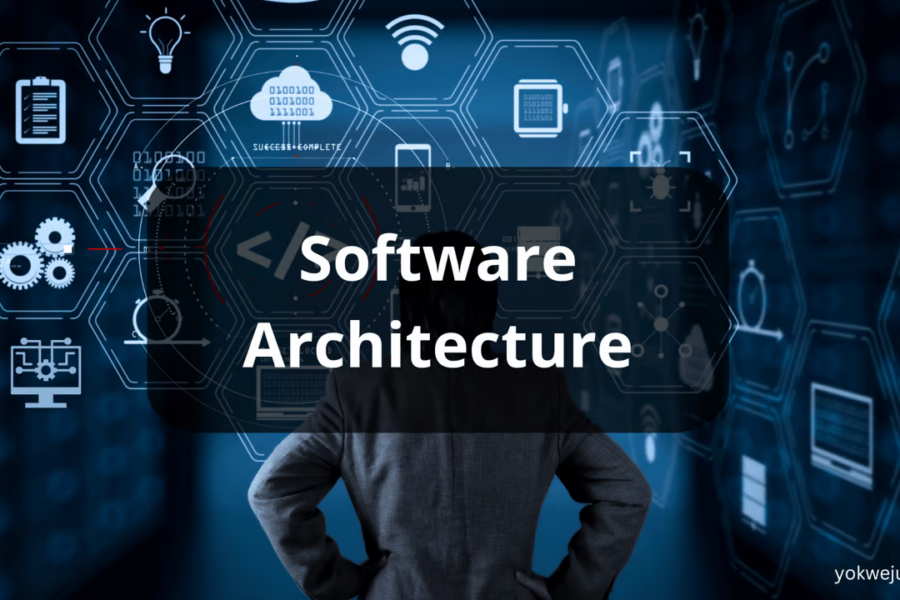
Conquer Your Software Architecture Journey: A Guided Exploration
Feeling overwhelmed by the vast landscape of software architecture resources? You’re not alone. Choosing the right books, articles, and roadmaps can be a daunting task. This post is here to help! This community thread on r/softwarearchitecture serves as a treasure trove of curated recommendations from experienced professionals. It tackles the age-old question: “What resources can…
Conquering the Cloud with Terraform: A 12-Day Roadmap
Terraform is an infrastructure as code (IaC) tool that allows you to define and provision your infrastructure in a safe, efficient, and repeatable way. This twelve-day roadmap, designed by Tech Fusionist, is your comprehensive guide to learning Terraform and becoming an infrastructure automation pro. Week 1: Terraform Foundations and Cloud Platform Integration Week 2: Advanced…
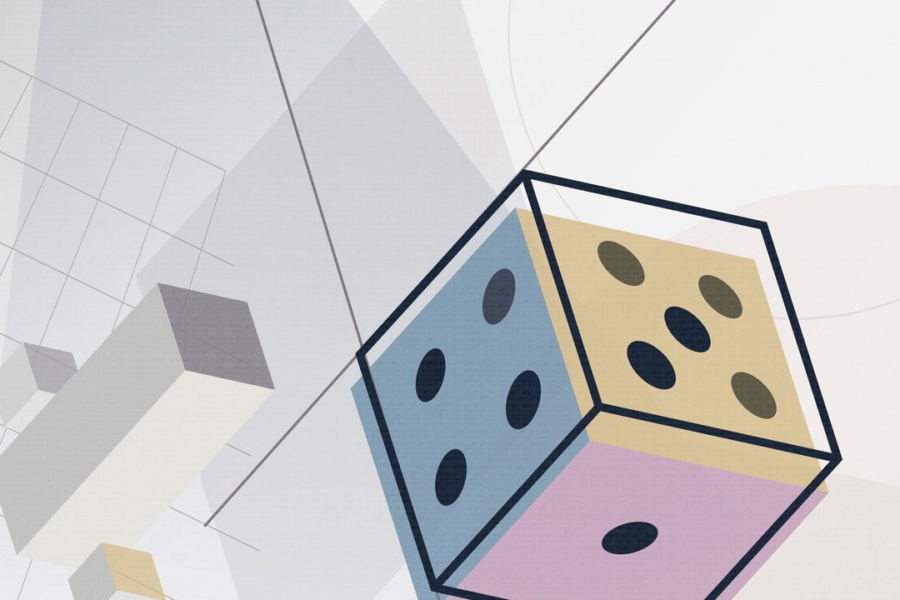
Demystifying the World with Probability: Introduction to Harvard’s Stat 110
Have you ever wondered how statistics help us understand the world around us? From weather forecasts to financial markets, probability plays a crucial role in making sense of randomness and uncertainty. If you’re looking for a solid foundation in this essential concept, then Harvard University’s Stat 110: Probability course might be the perfect place to…
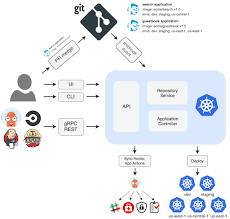
Optimize Your DevOps: Explore Codefresh’s Powerful New Tools
Date : June 11Time : 9-11 am PDT Are you exhausted from endlessly writing scripts, configuring CI/CD pipelines, and copying and pasting to promote changes between environments? Codefresh has a solution that will revolutionize your workflow. We are thrilled to introduce Environments and Products, designed to simplify and streamline the process of promoting changes across…