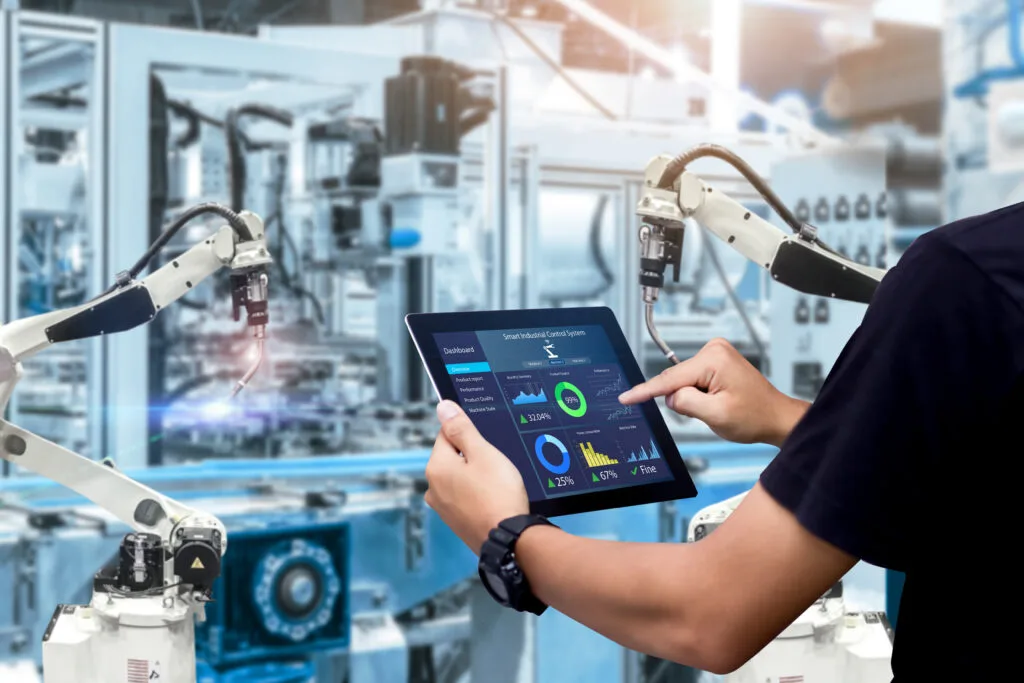
In today’s hyperconnected digital world, IT infrastructure is the foundation of business continuity and service delivery. From cloud-native applications to data centers and edge networks, organizations rely on a sprawling ecosystem of systems and hardware to keep operations running smoothly. As this infrastructure grows in complexity, so does the challenge of maintaining its health and availability. Traditional maintenance approaches—reactive or scheduled—are no longer sufficient. This is where Artificial Intelligence (AI) steps in, enabling predictive maintenance to anticipate problems before they impact operations.
What is Predictive Maintenance in IT?
Predictive maintenance in IT refers to the use of AI and machine learning techniques to proactively monitor infrastructure health, detect anomalies, and forecast failures. It differs from reactive maintenance, which only addresses issues after they’ve occurred, and preventive maintenance, which is performed on a fixed schedule regardless of actual need. Predictive maintenance leverages data-driven insights to perform maintenance activities only when necessary, based on real-time signals and historical trends. This leads to reduced downtime, cost efficiency, and improved reliability across IT environments.
From Reactive to Predictive: The Evolution of Maintenance
Historically, IT teams would wait for a server or component to fail before taking action—often resulting in service interruptions. Later, preventive maintenance introduced routine checkups to reduce such risks, but it came with its own downsides: unnecessary replacements and labor costs. Predictive maintenance represents a smarter third wave. By using AI to analyze metrics, system logs, and usage behavior, it predicts when a failure is likely to occur and enables preemptive action, minimizing both waste and disruption. It strikes the perfect balance between cost-effectiveness and operational excellence.
How AI Powers Predictive Maintenance
The backbone of predictive maintenance is data. IT systems constantly generate logs, performance metrics, and telemetry data. AI models analyze this data to detect patterns and uncover subtle changes that may indicate an impending failure. For instance, a gradual increase in CPU temperature or memory errors can suggest a server is nearing hardware degradation. By learning from this data over time, machine learning models become more accurate in their predictions, delivering real-time risk scores and maintenance recommendations. This data-driven foresight enables IT teams to act before issues escalate.
The Predictive Maintenance Workflow
A typical AI-driven predictive maintenance workflow begins with continuous data collection from servers, storage systems, networking hardware, and software applications. This data is then cleaned, normalized, and enriched to create meaningful features. Machine learning algorithms analyze this information to detect anomalies and forecast failure points. When a potential issue is detected, the system can automatically trigger alerts, generate maintenance tickets, or even initiate auto-remediation workflows—such as migrating workloads or restarting services. Over time, the AI models refine themselves using feedback and new data, making predictions even more precise.
Key Business Benefits
The advantages of predictive maintenance go beyond technical efficiency—they directly impact business outcomes. Organizations benefit from reduced unplanned downtime, which translates to better service availability and customer satisfaction. Maintenance becomes more strategic and less resource-intensive, cutting down on operational costs. Equipment life is extended through timely interventions, and teams can focus on strategic initiatives rather than reacting to emergencies. Moreover, meeting SLAs becomes easier when systems perform reliably, helping businesses maintain trust and compliance with customers and regulators alike.
Real-World Applications and Use Cases
In real-world IT operations, predictive maintenance is already making a tangible difference. Data centers use it to monitor cooling systems, power supplies, and storage arrays, allowing technicians to act before any disruption occurs. Cloud providers apply predictive analytics to their infrastructure to automatically move workloads from underperforming or soon-to-fail virtual machines. Network operators leverage AI to predict congestion or outages based on traffic and device metrics. Even in edge computing environments, where manual maintenance is impractical, AI ensures proactive care through autonomous predictions and actions.
Challenges and Considerations
While the promise of predictive maintenance is substantial, successful implementation requires careful planning. The accuracy of predictions depends heavily on data quality—poor or inconsistent data can lead to false positives or missed warnings. Integrating AI tools with existing IT monitoring and service management platforms may require customization. Additionally, skilled personnel are needed to train, fine-tune, and interpret machine learning models. Despite these challenges, with the right strategy and partners, organizations can unlock immense value and resilience from predictive maintenance solutions.
Conclusion
AI-powered predictive maintenance is reshaping the way businesses manage IT infrastructure. By shifting from reactive problem-solving to proactive foresight, organizations can dramatically improve uptime, efficiency, and cost-effectiveness. As AI continues to evolve and integrate with AIOps platforms, predictive maintenance will become an essential component of resilient digital operations. In a world where every second of downtime can mean lost revenue or customer trust, the ability to anticipate and prevent failures is a game-changer. The future of IT maintenance is predictive, intelligent, and powered by AI.
Follow us for more Updates